While AI won't replace lab experiments or human expertise any time soon, it has the potential to significantly enhance drug discovery. However, overcoming key challenges like data sparsity, lack of diversity, and inconsistencies in datasets is crucial. At Kvantify, we believe our physics-based models may offer a solution.
The impact of AI on drug discovery is a topic of a growing and often very polarized debate. Here it is important to remember that we tend to overestimate short-term impact and underestimate long-term achievements for all new technologies. A couple of examples:
- AI will not replace lab experiments. Quite the opposite: we see leading AI-driven drug discovery companies heavily investing in advanced lab equipment, especially in automation and robotics, to accelerate the design-make-test-analyze cycle. Labs are not going away. Instead, they are becoming much more efficient.
- AI will not replace human domain experts. As Alex Zhavoronkov from Insilico Medicine points out, medicinal chemists will likely be essential for steering the drug discovery process for at least the next twenty years. However, having access to better data is crucial for this decision-making, as it can help identify more promising drug candidates earlier in the process, lower failure rates in clinical trials, and reduce development costs.
- AI drugs are not failing in the clinic. The issue here is that there really is no such thing as an AI drug. The so-called AI drugs we have seen in clinical trials were developed using a variety of techniques, including human-led decision making, traditional lab validation methods, and classical computational approaches, such as physics-based modeling and simple regression and classification models. And since we have so few data points here, it is very hard to attribute a root cause for the failure of the few AI drugs that have made it into the clinic so far - and in general all methods have very high failure rates, making it even more difficult to measure any relative improvement. It is still too early to assess the short-term impact.
- Generative AI methods fail to generalize and tend to hallucinate. While this is true to a certain extent, all models have limitations and domains of applicability. This also goes for classical methods such as physics-based modelling: most force fields will happily provide you with an answer or a low-energy conformation - but we are still relying on approximations. However, one key distinction is that modern deep learning models fit millions or even billions of trainable parameters, potentially enabling them to capture more sophisticated patterns. In contrast, traditional methods fit much fewer parameters to theoretically grounded functional forms. So while deep learning offers greater expressiveness, the lack of constraints also introduces a higher risk of generalization error. Fortunately, we're starting to see better datasets, such as PLINDER and PoseBusters, with improved training-test splits, that will help us understand and quantify generalization.
But while there are pros and cons, there is no doubt that machine learning and AI can bring real value. DeepMind’s AlphaFold model surpassed all previous models and has provided us with an impressive catalog of structures for pretty much all types of proteins. And while not perfect, AlphaFold certainly raised the bar for what is possible in terms of modelling proteins.
Key Challenges for AI in Drug Discovery
While AI won't replace lab experiments or human expertise any time soon, it has the potential to significantly enhance data-driven decision-making and accelerate drug development. But while all this sounds promising, there are still some key challenges for AI to succeed in the life sciences:
- Sparsity of labelled data: In general, we have lots of data in biology. But while some unsupervised methods have been able to do impressive things using raw protein sequence data, there is a lack of publicly available data labelled with e.g. binding rate constants or mode-of-action. For instance, it is very hard to find unbinding kinetics data - something that led us to develop Koffee, the first commercial physics-based model for unbinding kinetics. Training a ML model here would simply not be feasible due to the lack of data.
- Lack of data diversity. Recently, Leash Bio garnered significant attention by releasing a massive dataset (300 million data points) in a Kaggle competition. Despite more than 2,000 submissions, none of the teams could develop a model that generalized well to new chemical scaffolds. Although the exact reason is unclear, one possible explanation is the lack of diversity in the training data. All the training data was derived from a single chemical scaffold, while the final test set was based on a different scaffold. Machine learning models will struggle when all of the training data contains a feature that is constant across all training data. Lack of data diversity is common and may take many different shapes - for instance, pharma companies may have quite extensive in-house datasets, but they are often limited to relatively few protein targets.
- Lack of uniform data. Even when large public datasets exist, they are often assembled from a mix of different experiments and measurements. Take ChEMBL, for instance—it provides a wealth of affinity data, but this data is expressed through various metrics (IC50, XC50, EC50, AC50, Ki, Kd, etc.) and obtained under different experimental conditions. This lack of uniformity makes it harder to train accurate models. A model would either have to learn the experimental differences or simply ignore them.
- Hard to validate. Even though many AI models now offer confidence scores and other reliability measures, it is still hard to know when the results can be trusted.
How can Kvantify help?
Since the inception, Kvantify has explored how far we can push physics-based models while keeping computational costs manageable. We believe our tools can handle some of the above challenges.
Not only are our physics-based models a great way to validate and verify the output of AI models - for instance you could run machine learning-based virtual screening, and then run the top-ranked hits through a physics-based model to confirm them before doing lab experiments.
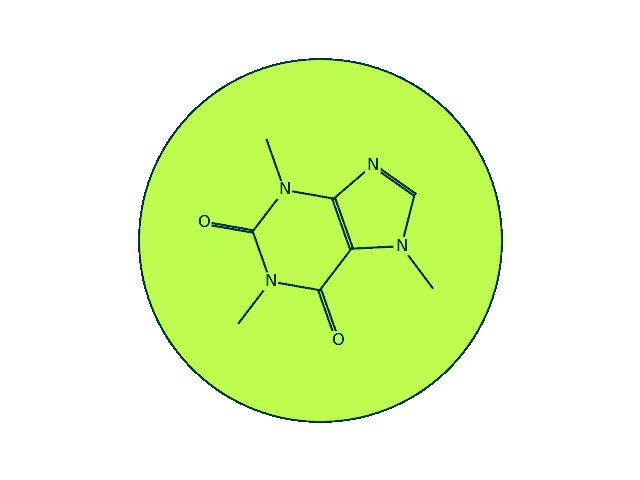
Our physics-based methods are also fast enough that we can generate additional data points that can be used when training models. This could, for instance, be models for elucidating structure-activity relations, if experimental data is sparse or not accessible. There are also no issues with inconsistency of data coming from different sources or measurement types.
We have also shown that it is possible to go even further and apply quantum chemistry to parts of a system - performing the most sensitive part of the calculations on a quantum computer. And while we are not there yet, the unique scaling of quantum chemistry problems on quantum computers will eventually enable us to tackle calculations that are simply impossible on classical computers.
Our first product, Koffee, demonstrated that our models can address the AI challenges mentioned above. We are soon ready to reveal what is coming next! Stay tuned.
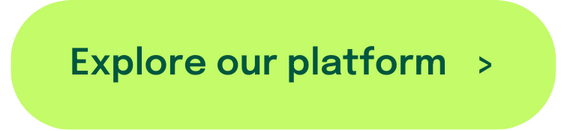